By: Oluwatosin Oyeladun
Technology advances in tandem with the world’s progress, and machine learning is a key component of this growth.
Machine learning has changed various sectors, giving humans never know before access into data analysis and decision making, but as we continue to finetune these machines learning models, their complexity continue to increase, this has brought out a new term called “Black Box” referring to models that make accurate predictions without giving credible insight into how the predictions were made.
In certain sector, such as healthcare, finance, security and many more, the margin to make errors are very slim and as such lack of interpretation or machine learning model results that cant be analysed properly can be very problematic.
In this article we will uncover advanced techniques for making machine learning models interpretable, ensuring their reliability and accuracy in critical areas and applications.
Our society heavily relies on the unbiased and credibility of certain sectors such as healthcare, finance, criminal justice, and autonomous systems and because of that, the consequences of incorrect or bias decisions can be heavier, affecting people and their livelihoods.
For example, in healthcare, machine learning models assist in diagnosing diseases and recommending treatments. In finance, they detect fraudulent transactions and assess credit risk. The inability to explain these decisions can lead to mistrust and potentially harmful outcomes.
To address these challenges, data scientists employ various methods to interpret and explain machine learning models. These methods provide insights into how models make decisions, ensuring transparency and accountability.
Feature importance methods identify which input variables most significantly impact the model’s predictions. Techniques such as SHAP (SHapley Additive exPlanations) and LIME (Local Interpretable Model-agnostic Explanations) are widely used to quantify the contribution of each feature. SHAP values provide a unified measure of feature importance, offering consistency across different models. LIME explains individual predictions by approximating the model locally with an interpretable model, such as a linear regression.
Surrogate models are simpler, interpretable models that approximate the behavior of complex models. Decision trees or linear models often serve as surrogates.Data scientists can learn about the patterns and decision rules by training a surrogate model to replicate the black-box model’s predictions. When the primary model is too complex for direct interpretation, this method is especially helpful.
Visualisation tools help data scientists and stakeholders understand the relationships between features and predictions. Partial dependence plots (PDPs) show the effect of one or two features on the predicted outcome, averaged over the dataset. Individual conditional expectation (ICE) plots provide a more detailed view by showing how predictions change with varying feature values for individual data points. These visualisations make complex models more accessible and understandable.
Rule extraction techniques derive human-readable rules from complex models. Methods like decision tree induction or rule-based systems transform the black-box model into a set of if-then rules. These rules are easier to interpret and can be validated against domain knowledge. This technique is particularly useful in regulatory environments where explicit decision rules are required.
The push for interpretable machine learning is driven by real-world needs. For instance, in healthcare, interpretable models can help clinicians understand and trust AI recommendations. In finance, regulators require transparency to ensure fair lending practices. Data scientists must balance model complexity with interpretability to meet these demands.
Despite the progress in interpretability, challenges remain. Complex models, such as deep neural networks, are inherently difficult to interpret. Balancing accuracy and interpretability is an ongoing struggle. Data scientists must also ensure that interpretability methods do not introduce biases or inaccuracies.
The future of interpretable machine learning lies in developing interpretable models that do not sacrifice performance. Research is ongoing to create models that are both accurate and transparent from the outset.
Machine learning models need to be unbias and easy to interpret particularly for high-stake decision making sectors to win users trust and ensure transparency.
By employing techniques that help reduce bias and and easily extract the data, data scientists can unlock the black box and provide valuable insights into model behavior.
These developments are essential for fostering responsibility and confidence in fields where choices have broad ramifications. The goal of interpretability will continue to be a primary priority as data science develops, spurring innovation and guaranteeing the responsible application of AI technology.
About the writer:
Oluwatosin Oyeladun is an African voice in the machine learning field. Oyeladun has delivered numerous multimillion-dollar projects that have disrupted the African digital payments status quo. His leadership in machine learning and data science has enabled development and deployment of AI algorithms that massively reduces fraud exposure for millions of users across Africa, enabling them to transact safely and effortlessly. Oyeladun has arguably raised the bar on technology innovation more generally.
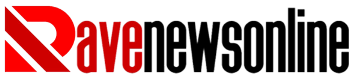